Introduction to Python
Contents
Introduction to Python¶
Before we get started …¶
most of what you’ll see within this lecture was prepared by Ross Markello, Michael Notter and Peer Herholz and further adapted for this course by Peer Herholz
based on Tal Yarkoni’s “Introduction to Python” lecture at Neurohackademy 2019
based on http://www.stavros.io/tutorials/python/ & http://www.swaroopch.com/notes/python
based on https://github.com/oesteban/biss2016 & https://github.com/jvns/pandas-cookbook
Goals¶
learn basic and efficient usage of the python programming language
what is python & how to utilize it
building blocks of & operations in python
What is Python?¶
Python is a programming language
Specifically, it’s a widely used, very flexible, high-level, general-purpose, dynamic programming language
That’s a mouthful! Let’s explore each of these points in more detail…
Widely-used¶
Python is the fastest-growing major programming language
Top 3 overall (with JavaScript, Java)

High-level¶
Python features a high level of abstraction
Many operations that are explicit in lower-level languages (e.g., C/C++) are implicit in Python
E.g., memory allocation, garbage collection, etc.
Python lets you write code faster
File reading in Java¶
import java.io.BufferedReader;
import java.io.FileReader;
import java.io.IOException;
public class ReadFile {
public static void main(String[] args) throws IOException{
String fileContents = readEntireFile("./foo.txt");
}
private static String readEntireFile(String filename) throws IOException {
FileReader in = new FileReader(filename);
StringBuilder contents = new StringBuilder();
char[] buffer = new char[4096];
int read = 0;
do {
contents.append(buffer, 0, read);
read = in.read(buffer);
} while (read >= 0);
return contents.toString();
}
}
General-purpose¶
You can do almost everything in Python
Comprehensive standard library
Enormous ecosystem of third-party packages
Widely used in many areas of software development (web, dev-ops, data science, etc.)
Dynamic¶
Code is interpreted at run-time
No compilation process*; code is read line-by-line when executed
Eliminates delays between development and execution
The downside: poorer performance compared to compiled languages
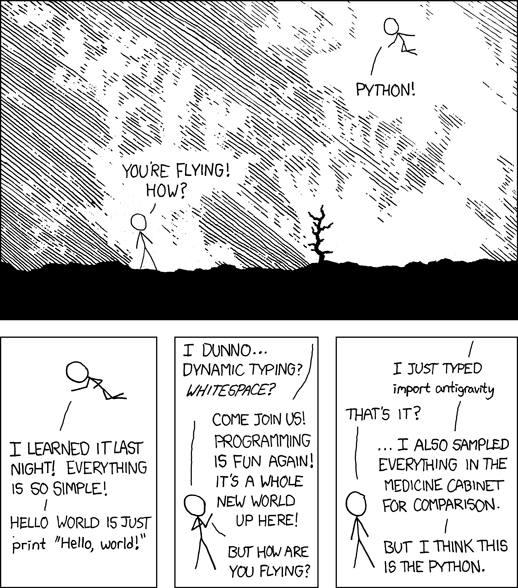
(Try typing import antigravity
into a new cell and running it!)
Variables and data types¶
In Python, we declare a variable by assigning it a value with the = sign
Variables are pointers, not data stores!
Python supports a variety of data types and structures:
booleans
numbers (ints, floats, etc.)
strings
lists
dictionaries
many others!
We don’t specify a variable’s type at assignment
What follows is a short introduction to Python
to help beginners to get familiar with this programming language
.
It is divided into the following chapters:
Module¶
Most of the functionality in Python is provided by modules. To use a module in a Python program it first has to be imported. A module can be imported using the import
statement. For example, to import the module math
, which contains many standard mathematical functions, we can do:
import math
This includes the whole module and makes it available for use later in the program. For example, we can do:
import math
x = math.cos(2 * math.pi)
print(x)
1.0
Importing the whole module us often times unnecessary and can lead to longer loading time or increase the memory consumption. An alternative to the previous method, we can also choose to import only a few selected functions from a module by explicitly listing which ones we want to import:
from math import cos, pi
x = cos(2 * pi)
print(x)
1.0
It is also possible to give an imported module or symbol your own access name with the as
additional:
import numpy as np
from math import pi as number_pi
x = np.rad2deg(number_pi)
print(x)
180.0
Namespaces and imports¶
Python is very serious about maintaining orderly namespaces
If you want to use some code outside the current scope, you need to explicitly “import” it
Python’s import system often annoys beginners, but it substantially increases code clarity
Almost completely eliminates naming conflicts and confusion
Help and Descriptions¶
Using the function help
we can get a description of almost all functions.
help(math.log)
Help on built-in function log in module math:
log(...)
log(x, [base=math.e])
Return the logarithm of x to the given base.
If the base not specified, returns the natural logarithm (base e) of x.
math.log(10)
2.302585092994046
math.log(10, 2)
3.3219280948873626
Variables and types¶
Symbol names¶
Variable names in Python can contain alphanumerical characters a-z
, A-Z
, 0-9
and some special characters such as _
. Normal variable names must start with a letter.
By convention, variable names start with a lower-case letter, and Class names start with a capital letter.
In addition, there are a number of Python keywords that cannot be used as variable names. These keywords are:
and, as, assert, break, class, continue, def, del, elif, else, except, exec, finally, for, from, global, if, import, in, is, lambda, not, or, pass, print, raise, return, try, while, with, yield
Assignment¶
The assignment operator in Python is =
. Python is a dynamically typed language, so we do not need to specify the type of a variable when we create one.
Assigning a value to a new variable creates the variable:
# variable assignments
x = 1.0
Although not explicitly specified, a variable does have a type associated with it. The type is derived from the value it was assigned.
type(x)
float
If we assign a new value to a variable, its type can change.
x = 1
type(x)
int
If we try to use a variable that has not yet been defined we get an NameError
(Note, that we will use in the notebooks try/except
blocks to handle the exception, so the notebook doesn’t stop. The code below will try to execute print
function and if the NameError
occurs the error message will be printed. Otherwise, an error will be raised. Later in this notebook you will learn more about exception handling.):
try:
print(y)
except(NameError) as err:
print("NameError", err)
else:
raise
NameError name 'y' is not defined
Variable names:
Can include letters, digits, and underscores
Cannot start with a digit
Are case sensitive.
This means that, for example:
weight0
is a valid variable name, whereas0weight
is notweight
andWeight
are different variables
Fundamental types & data structures¶
Most code requires more complex structures built out of basic data types
Python provides built-in support for many common structures
Many additional structures can be found in the collections module
# integers
x = 1
type(x)
int
# float
x = 1.0
type(x)
float
# boolean
b1 = True
b2 = False
type(b1)
bool
# string
s = "hello world"
type(s)
str
Operators and comparisons¶
Most operators and comparisons in Python work as one would expect:
Arithmetic operators
+
,-
,*
,/
,**
power,%
modulo
[1 + 2,
1 - 2,
1 * 2,
1 % 2]
[3, -1, 2, 1]
In Python 2.7, what kind of division (/
) will be executed, depends on the type of the numbers involved. If all numbers are integers, the division will be an integer division, otherwise, it will be a float division. In Python 3 this has been changed and fractions aren’t lost when dividing integers (for integer division you can use another operator, //
).
# In Python 3 these two operations will give the same result
# (in Python 2 the first one will be treated as an integer division).
print(1 / 2)
print(1 / 2.0)
0.5
0.5
# Note! The power operator in python isn't ^, but **
2 ** 2
4
The boolean operators are spelled out as words
and
,not
,or
.
True and False
False
not False
True
True or False
True
Comparison operators
>
,<
,>=
(greater or equal),<=
(less or equal),==
(equal),!=
(not equal) andis
(identical).
2 > 1, 2 < 1
(True, False)
2 > 2, 2 < 2
(False, False)
2 >= 2, 2 <= 2
(True, True)
# equal to
[1,2] == [1,2]
True
# not equal to
2 != 3
True
boolean operator
x = True
y = False
print(not x)
print(x and y)
print(x or y)
False
False
True
String comparison
"lo W" in "Hello World"
True
"x" not in "Hello World"
True
Shortcut math operation and assignment¶
a = 2
a = a * 2
print(a)
4
The command a = a * 2
, can be shortcut to a *= 2
. This also works with +=
, -=
and /=
.
b = 3
b *= 3
print(b)
9
Strings, List and dictionaries¶
Strings¶
Strings are the variable type that is used for storing text messages.
s = "Hello world"
type(s)
str
# length of the string: number of characters in string
len(s)
11
# replace a substring in a string with something else
s2 = s.replace("world", "test")
print(s2)
Hello test
We can index a character in a string using []
:
s[0]
'H'
Heads up MATLAB users: Indexing start at 0!
We can extract a part of a string using the syntax [start:stop]
, which extracts characters between index start
and stop
:
s[0:5]
'Hello'
If we omit either (or both) of start
or stop
from [start:stop]
, the default is the beginning and the end of the string, respectively:
s[:5]
'Hello'
s[6:]
'world'
s[:]
'Hello world'
We can also define the step size using the syntax [start:end:step]
(the default value for step
is 1, as we saw above):
s[::1]
'Hello world'
s[::2]
'Hlowrd'
This technique is called slicing.
String formatting examples¶
print("str1" + "str2" + "str3") # strings added with + are concatenated without space
str1str2str3
print("str1" "str2" "str3") # The print function concatenates strings differently
print("str1", "str2", "str3") # depending on how the inputs are specified
print(("str1", "str2", "str3")) # See the three different outputs below
str1str2str3
str1 str2 str3
('str1', 'str2', 'str3')
print("str1", 1.0, False) # The print function converts all arguments to strings
str1 1.0 False
print("value = %f" %1.0) # we can use C-style string formatting
value = 1.000000
Python has two string formatting styles. An example of the old style is below, specifier %.2f
transforms the input number into a string, that corresponds to a floating point number with 2 decimal places and the specifier %d
transforms the input number into a string, corresponding to a decimal number.
s2 = "value1 = %.2f. value2 = %d" % (3.1415, 1.5)
print(s2)
value1 = 3.14. value2 = 1
The same string can be written using the new style string formatting.
s3 = 'value1 = {:.2f}, value2 = {}'.format(3.1415, 1.5)
print(s3)
value1 = 3.14, value2 = 1.5
print("Newlines are indicated by \nAnd tabs by \t.")
print(r"Newlines are indicated by \nAnd tabs by \t. Printed as rawstring")
Newlines are indicated by
And tabs by .
Newlines are indicated by \nAnd tabs by \t. Printed as rawstring
print("Name: {}\nNumber: {}\nString: {}".format("Nipype", 3, 3 * "-"))
Name: Nipype
Number: 3
String: ---
strString = """This is
a multiline
string."""
print(strString)
This is
a multiline
string.
print("This {verb} a {noun}.".format(noun = "test", verb = "is"))
This is a test.
Single Quote¶
You can specify strings using single quotes such as 'Quote me on this'
.
All white space i.e. spaces and tabs, within the quotes, are preserved as-is.
Double Quotes¶
Strings in double quotes work exactly the same way as strings in single quotes. An example is "What's your name?"
.
Triple Quotes¶
You can specify multi-line strings using triple quotes - ("""
or '''
). You can use single quotes and double quotes freely within the triple quotes. An example is:
'''This is a multi-line string. This is the first line.
This is the second line.
"What's your name?," I asked.
He said "Bond, James Bond."
'''
'This is a multi-line string. This is the first line.\nThis is the second line.\n"What\'s your name?," I asked.\nHe said "Bond, James Bond."\n'
List¶
Lists are very similar to strings, except that each element can be of any type.
The syntax for creating lists in Python is [...]
:
l = [1,2,3,4]
print(type(l))
print(l)
<class 'list'>
[1, 2, 3, 4]
We can use the same slicing techniques to manipulate lists as we could use on strings:
print(l)
print(l[1:3])
print(l[::2])
[1, 2, 3, 4]
[2, 3]
[1, 3]
Heads up MATLAB users: Indexing starts at 0!
l[0]
1
Elements in a list do not all have to be of the same type:
l = [1, 'a', 1.0]
print(l)
[1, 'a', 1.0]
Python lists can be inhomogeneous and arbitrarily nested:
nested_list = [1, [2, [3, [4, [5]]]]]
nested_list
[1, [2, [3, [4, [5]]]]]
Lists play a very important role in Python and are for example used in loops and other flow control structures (discussed below). There are a number of convenient functions for generating lists of various types, for example, the range
function (note that in Python 3 range
creates a generator, so you have to use list
function to get a list):
start = 10
stop = 30
step = 2
list(range(start, stop, step))
[10, 12, 14, 16, 18, 20, 22, 24, 26, 28]
# convert a string to a list by type casting:
print(s)
s2 = list(s)
s2
Hello world
['H', 'e', 'l', 'l', 'o', ' ', 'w', 'o', 'r', 'l', 'd']
# sorting lists
s2.sort()
print(s2)
[' ', 'H', 'd', 'e', 'l', 'l', 'l', 'o', 'o', 'r', 'w']
Adding, inserting, modifying, and removing elements from lists¶
# create a new empty list
l = []
# add an elements using `append`
l.append("A")
l.append("d")
l.append("d")
print(l)
['A', 'd', 'd']
We can modify lists by assigning new values to elements in the list. In technical jargon, lists are mutable.
l[1] = "p"
l[2] = "t"
print(l)
['A', 'p', 't']
l[1:3] = ["s", "m"]
print(l)
['A', 's', 'm']
Insert an element at an specific index using insert
l.insert(0, "i")
l.insert(1, "n")
l.insert(2, "s")
l.insert(3, "e")
l.insert(4, "r")
l.insert(5, "t")
print(l)
['i', 'n', 's', 'e', 'r', 't', 'A', 's', 'm']
Remove first element with specific value using ‘remove’
l.remove("A")
print(l)
['i', 'n', 's', 'e', 'r', 't', 's', 'm']
Remove an element at a specific location using del
:
del l[7]
del l[6]
print(l)
['i', 'n', 's', 'e', 'r', 't']
Lists¶
An ordered, heterogeneous collection of objects
List elements can be accessed by position
Tuples¶
Tuples are like lists, except that they cannot be modified once created, that is they are immutable.
In Python, tuples are created using the syntax (..., ..., ...)
, or even ..., ...
:
point = (10, 20)
print(type(point))
print(point)
<class 'tuple'>
(10, 20)
If we try to assign a new value to an element in a tuple we get an error:
try:
point[0] = 20
except(TypeError) as er:
print("TypeError:", er)
else:
raise
TypeError: 'tuple' object does not support item assignment
Tuples¶
Very similar to lists
Key difference: tuples are immutable
They can’t be modified once they’re created
Dictionaries¶
Dictionaries are also like lists, except that each element is a key-value pair. The syntax for dictionaries is {key1 : value1, ...}
:
params = {"parameter1" : 1.0,
"parameter2" : 2.0,
"parameter3" : 3.0,}
print(type(params))
print(params)
<class 'dict'>
{'parameter1': 1.0, 'parameter2': 2.0, 'parameter3': 3.0}
Dictionary entries can only be accessed by their key name.
params["parameter2"]
2.0
print("parameter1 = " + str(params["parameter1"]))
print("parameter2 = " + str(params["parameter2"]))
print("parameter3 = " + str(params["parameter3"]))
parameter1 = 1.0
parameter2 = 2.0
parameter3 = 3.0
params["parameter1"] = "A"
params["parameter2"] = "B"
# add a new entry
params["parameter4"] = "D"
print("parameter1 = " + str(params["parameter1"]))
print("parameter2 = " + str(params["parameter2"]))
print("parameter3 = " + str(params["parameter3"]))
print("parameter4 = " + str(params["parameter4"]))
parameter1 = A
parameter2 = B
parameter3 = 3.0
parameter4 = D
Dictionaries (dict)¶
Unordered collection of key-to-value pairs
dictionary elements can be accessed by key, but not by position
Everything is an object in Python¶
All of these ‘data types’ are actually just objects in Python
Everything is an object in Python!
The operations you can perform with a variable depend on the object’s definition
E.g., the multiplication operator * is defined for some objects but not others
Indentation¶
Whitespace is important in Python. Actually, whitespace at the beginning of the line is important. This is called indentation. Leading whitespace (spaces and tabs) at the beginning of the logical line is used to determine the indentation level of the logical line, which in turn is used to determine the grouping of statements.
This means that statements which go together must have the same indentation, for example:
i = 5
print('Value is ', i)
print('I repeat, the value is ', i)
Value is 5
I repeat, the value is 5
Each such set of statements is called a block. We will see examples of how blocks are important later on.
One thing you should remember is that wrong indentation rises IndentationError
.
Control Flow¶
Control structures¶
Language features that allow us to control how code is executed
Iteration (e.g., for-loops, while statements…)
Conditionals (if-then-else statements)
Etc…
Conditional statements: if, elif, else¶
The Python syntax for conditional execution of code use the keywords if
, elif
(else if), else
:
statement1 = False
statement2 = False
if statement1:
print("statement1 is True")
elif statement2:
print("statement2 is True")
else:
print("statement1 and statement2 are False")
statement1 and statement2 are False
For the first time, here we encountered a peculiar and unusual aspect of the Python programming language: Program blocks are defined by their indentation level. In Python, the extent of a code block is defined by the indentation level (usually a tab or say four white spaces). This means that we have to be careful to indent our code correctly, or else we will get syntax errors.
Examples:
# Good indentation
statement1 = statement2 = True
if statement1:
if statement2:
print("both statement1 and statement2 are True")
both statement1 and statement2 are True
# Bad indentation! This would lead to error
#if statement1:
# if statement2:
# print("both statement1 and statement2 are True") # this line is not properly indented
statement1 = False
if statement1:
print("printed if statement1 is True")
print("still inside the if block")
if statement1:
print("printed if statement1 is True")
print("now outside the if block")
now outside the if block
Loops¶
In Python, loops can be programmed in a number of different ways. The most common is the for
loop, which is used together with iterable objects, such as lists. The basic syntax is:
for
loops¶
for x in [1,2,3]:
print(x),
1
2
3
The for
loop iterates over the elements of the supplied list and executes the containing block once for each element. Any kind of list can be used in the for
loop. For example:
for x in range(4): # by default range start at 0
print(x),
0
1
2
3
Note: range(4)
does not include 4 !
for x in range(-3,3):
print(x),
-3
-2
-1
0
1
2
for word in ["scientific", "computing", "with", "python"]:
print(word)
scientific
computing
with
python
To iterate over key-value pairs of a dictionary:
for key, value in params.items():
print(key + " = " + str(value))
parameter1 = A
parameter2 = B
parameter3 = 3.0
parameter4 = D
Sometimes it is useful to have access to the indices of the values when iterating over a list. We can use the enumerate
function for this:
for idx, x in enumerate(range(-3,3)):
print(idx, x)
0 -3
1 -2
2 -1
3 0
4 1
5 2
break
, continue
and pass
¶
To control the flow of a certain loop you can also use break
, continue
and pass
.
rangelist = list(range(10))
print(list(rangelist))
for number in rangelist:
# Check if number is one of
# the numbers in the tuple.
if number in [4, 5, 7, 9]:
# "Break" terminates a for without
# executing the "else" clause.
break
else:
# "Continue" starts the next iteration
# of the loop. It's rather useless here,
# as it's the last statement of the loop.
print(number)
continue
else:
# The "else" clause is optional and is
# executed only if the loop didn't "break".
pass # Do nothing
[0, 1, 2, 3, 4, 5, 6, 7, 8, 9]
0
1
2
3
List comprehensions: Creating lists using for
loops:
A convenient and compact way to initialize lists:
l1 = [x**2 for x in range(0,5)]
print(l1)
[0, 1, 4, 9, 16]
while
loops:
i = 0
while i < 5:
print(i)
i = i + 1
print("done")
0
1
2
3
4
done
Note that the print "done"
statement is not part of the while
loop body because of the difference in the indentation.
Functions¶
A function in Python is defined using the keyword def
, followed by a function name, a signature within parentheses ()
, and a colon :
. The following code, with one additional level of indentation, is the function body.
def say_hello():
# block belonging to the function
print('hello world')
say_hello() # call the function
hello world
Functions¶
A block of code that only runs when explicitly called
Can accept arguments (or parameters) that alter its behavior
Can accept any number/type of inputs, but always return a single object
Note: functions can return tuples (may look like multiple objects)
Following an example where we also feed two arguments into the function.
def print_max(a, b):
if a > b:
print( a, 'is maximum')
elif a == b:
print(a, 'is equal to', b)
else:
print(b, 'is maximum')
# directly pass literal values
print_max(3, 4)
x = 7
y = 7
# pass variables as arguments
print_max(x, y)
4 is maximum
7 is equal to 7
Very important: Variables inside a function are treated as local variables and therefore don’t interfere with variables outside the scope of the function.
x = 50
def func(x):
print('x is', x)
x = 2
print('Changed local x to', x)
func(x)
print('x is still', x)
x is 50
Changed local x to 2
x is still 50
The local scope of a variable inside a function can be extended with the keyword global
.
x = 50
def func():
global x
print('x is', x)
x = 2
print('Changed global x to', x)
func()
print('Value of x is', x)
x is 50
Changed global x to 2
Value of x is 2
Optionally, but highly recommended, we can define a so called “docstring”, which is a description of the functions purpose and behavior. The docstring should follow directly after the function definition, before the code in the function body.
def func1(s):
"""
Print a string 's' and tell how many characters it has
"""
print(s + " has " + str(len(s)) + " characters")
help(func1)
Help on function func1 in module __main__:
func1(s)
Print a string 's' and tell how many characters it has
func1("test")
test has 4 characters
Functions that return a value use the return
keyword:
def square(x):
"""
Return the square of x.
"""
return x ** 2
square(4)
16
We can return multiple values from a function using tuples (see above):
def powers(x):
"""
Return a few powers of x.
"""
return x ** 2, x ** 3, x ** 4
powers(3)
(9, 27, 81)
And if we know that a function returns multiple outputs, we can store them directly in multiple variables.
x2, x3, x4 = powers(3)
print(x3)
27
Positional vs. keyword arguments¶
Positional arguments are defined by position and must be passed
Arguments in the function signature are filled in order
Keyword arguments have a default value
Arguments can be passed in arbitrary order (after any positional arguments)
Default argument and keyword arguments¶
In a definition of a function, we can give default values to the arguments the function takes:
def myfunc(x, p=2, debug=False):
if debug:
print("evaluating myfunc for x = " + str(x) + " using exponent p = " + str(p))
return x**p
If we don’t provide a value of the debug
argument when calling the the function myfunc
it defaults to the value provided in the function definition:
myfunc(5)
25
myfunc(5, debug=True)
evaluating myfunc for x = 5 using exponent p = 2
25
If we explicitly list the name of the arguments in the function calls, they do not need to come in the same order as in the function definition. This is called keyword arguments and is often very useful in functions that take a lot of optional arguments.
myfunc(p=3, debug=True, x=7)
evaluating myfunc for x = 7 using exponent p = 3
343
*args
and *kwargs
parameters¶
Sometimes you might want to define a function that can take any number of parameters, i.e. variable number of arguments, this can be achieved by using one (*args
) or two (**kwargs
) asterisks in the function declaration. *args
is used to pass a non-keyworded, variable-length argument list and the **kwargs
is used to pass a keyworded, variable-length argument list.
def args_func(arg1, *args):
print("Formal arg:", arg1)
for a in args:
print("additioanl arg:", a)
args_func(1, "two", 3, [1, 2, 3])
Formal arg: 1
additioanl arg: two
additioanl arg: 3
additioanl arg: [1, 2, 3]
def kwargs_func(arg1, **kwargs):
print("kwargs is now a dictionary...\nType: %s\nContent: %s\n" % (type(kwargs), kwargs))
print("Formal arg:", arg1)
for key in kwargs:
print("another keyword arg: %s: %s" % (key, kwargs[key]))
kwargs_func(arg1=1, myarg2="two", myarg3=3)
kwargs is now a dictionary...
Type: <class 'dict'>
Content: {'myarg2': 'two', 'myarg3': 3}
Formal arg: 1
another keyword arg: myarg2: two
another keyword arg: myarg3: 3
Unnamed functions: lambda function¶
In Python we can also create unnamed functions, using the lambda
keyword:
f1 = lambda x: x**2
# is equivalent to
def f2(x):
return x**2
f1(2), f2(2)
(4, 4)
This technique is useful for example when we want to pass a simple function as an argument to another function, like this:
# map is a built-in python function
list(map(lambda x: x**2, range(-3,4)))
[9, 4, 1, 0, 1, 4, 9]
Classes¶
Classes are the key features of object-oriented programming. A class is a structure for representing an object and the operations that can be performed on the object.
In Python, a class can contain attributes (variables) and methods (functions).
A class is defined almost like a function, but using the class
keyword, and the class definition usually contains a number of class method definitions (a function in a class).
Each class method should have an argument
self
as it first argument. This object is a self-reference.Some class method names have special meaning, for example:
__init__
: The name of the method that is invoked when the object is first created.__str__
: A method that is invoked when a simple string representation of the class is needed, as for example when printed.There are many more, see http://docs.python.org/3.6/reference/datamodel.html#special-method-names
class Point:
"""
Simple class for representing a point in a Cartesian coordinate system.
"""
def __init__(self, x, y):
"""
Create a new Point at x, y.
"""
self.x = x
self.y = y
def translate(self, dx, dy):
"""
Translate the point by dx and dy in the x and y direction.
"""
self.x += dx
self.y += dy
def __str__(self):
return("Point at [%f, %f]" % (self.x, self.y))
To create a new instance of a class:
p1 = Point(0, 0) # this will invoke the __init__ method in the Point class
print(p1) # this will invoke the __str__ method
Point at [0.000000, 0.000000]
To invoke a class method in the class instance p
:
p2 = Point(1, 1)
print(p2)
p2.translate(0.25, 1.5)
print(p2)
Point at [1.000000, 1.000000]
Point at [1.250000, 2.500000]
You can access any value of a class object directly, for example:
print(p1.x)
p1.x = 10
print(p1)
0
Point at [10.000000, 0.000000]
Modules¶
One of the most important concepts in good programming is to reuse code and avoid repetitions.
The idea is to write functions and classes with a well-defined purpose and scope, and reuse these instead of repeating similar code in different part of a program (modular programming). The result is usually that readability and maintainability of a program are greatly improved. What this means in practice is that our programs have fewer bugs, are easier to extend and debug/troubleshoot.
Python supports modular programming at different levels. Functions and classes are examples of tools for low-level modular programming. Python modules are a higher-level modular programming construct, where we can collect related variables, functions, and classes in a module. A python module is defined in a python file (with file-ending .py
), and it can be made accessible to other Python modules and programs using the import
statement.
Consider the following example: the file mymodule.py
contains simple example implementations of a variable, function and a class:
%%file mymodule.py
"""
Example of a python module. Contains a variable called my_variable,
a function called my_function, and a class called MyClass.
"""
my_variable = 0
def my_function():
"""
Example function
"""
return my_variable
class MyClass:
"""
Example class.
"""
def __init__(self):
self.variable = my_variable
def set_variable(self, new_value):
"""
Set self.variable to a new value
"""
self.variable = new_value
def get_variable(self):
return self.variable
Writing mymodule.py
Note: %%file
is called a cell-magic function and creates a file that has the following lines as content.
We can import the module mymodule
into our Python program using import
:
import mymodule
Use help(module)
to get a summary of what the module provides:
help(mymodule)
Help on module mymodule:
NAME
mymodule
DESCRIPTION
Example of a python module. Contains a variable called my_variable,
a function called my_function, and a class called MyClass.
CLASSES
builtins.object
MyClass
class MyClass(builtins.object)
| Example class.
|
| Methods defined here:
|
| __init__(self)
| Initialize self. See help(type(self)) for accurate signature.
|
| get_variable(self)
|
| set_variable(self, new_value)
| Set self.variable to a new value
|
| ----------------------------------------------------------------------
| Data descriptors defined here:
|
| __dict__
| dictionary for instance variables (if defined)
|
| __weakref__
| list of weak references to the object (if defined)
FUNCTIONS
my_function()
Example function
DATA
my_variable = 0
FILE
/Users/peerherholz/google_drive/GitHub/DGPA_workshop_2022/workshop/prerequisites/mymodule.py
mymodule.my_variable
0
mymodule.my_function()
0
my_class = mymodule.MyClass()
my_class.set_variable(10)
my_class.get_variable()
10
If we make changes to the code in mymodule.py
, we need to reload it using reload
:
from importlib import reload
reload(mymodule)
<module 'mymodule' from '/Users/peerherholz/google_drive/GitHub/DGPA_workshop_2022/workshop/prerequisites/mymodule.py'>
Exceptions¶
In Python errors are managed with a special language construct called “Exceptions”. When errors occur exceptions can be raised, which interrupts the normal program flow and fallback to somewhere else in the code where the closest try-except statement is defined.
To generate an exception we can use the raise
statement, which takes an argument that must be an instance of the class BaseExpection
or a class derived from it.
try:
raise Exception("description of the error")
except(Exception) as err:
print ("Exception:", err)
Exception: description of the error
A typical use of exceptions is to abort functions when some error condition occurs, for example:
def my_function(arguments):
if not verify(arguments):
raise Exception("Invalid arguments")
# rest of the code goes here
To gracefully catch errors that are generated by functions and class methods, or by the Python interpreter itself, use the try
and except
statements:
try:
# normal code goes here
except:
# code for error handling goes here
# this code is not executed unless the code
# above generated an error
For example:
try:
print("test")
# generate an error: the variable test is not defined
print(test)
except:
print("Caught an exception")
test
Caught an exception
To get information about the error, we can access the Exception
class instance that describes the exception by using for example:
except Exception as e:
try:
print("test")
# generate an error: the variable test is not defined
print(test)
except Exception as e:
print("Caught an exception:" + str(e))
finally:
print("This block is executed after the try- and except-block.")
test
Caught an exception:name 'test' is not defined
This block is executed after the try- and except-block.
def some_function():
try:
# Division by zero raises an exception
10 / 0
except ZeroDivisionError:
print("Oops, invalid.")
else:
# Exception didn't occur, we're good.
pass
finally:
# This is executed after the code block is run
# and all exceptions have been handled, even
# if a new exception is raised while handling.
print("We're done with that.")
some_function()
Oops, invalid.
We're done with that.
You will see more exception handling examples in this and other notebooks.
File I/O¶
This section should give you a basic knowledge about how to read and write CSV or TXT files. First, let us create a CSV and TXT file about demographic information of 10 subjects (experiment_id, subject_id, gender, age).
%%file demographics.csv
ds102,sub001,F,21.94
ds102,sub002,M,22.79
ds102,sub003,M,19.65
ds102,sub004,M,25.98
ds102,sub005,M,23.24
ds102,sub006,M,23.27
ds102,sub007,D,34.72
ds102,sub008,D,22.22
ds102,sub009,M,22.7
ds102,sub010,D,25.24
Writing demographics.csv
%%file demographics.txt
ds102 sub001 F 21.94
ds102 sub002 M 22.79
ds102 sub003 M 19.65
ds102 sub004 M 25.98
ds102 sub005 M 23.24
ds102 sub006 M 23.27
ds102 sub007 D 34.72
ds102 sub008 D 22.22
ds102 sub009 M 22.7
ds102 sub010 D 25.24
Writing demographics.txt
Reading CSV files¶
Parsing comma-separated-values (CSV) files is a common task. There are many tools available in Python to deal with this. Let’s start by using the built-in csv
module.
import csv
Before you can read or write any kind of file, you first have to open the file and go through its content with a reader function or write the output line by line with a write function.
f = open('demographics.csv','r') # open the file with reading rights = 'r'
data = [i for i in csv.reader(f) ] # go through file and read each line
f.close() # close the file again
for line in data:
print(line)
['ds102', 'sub001', 'F', '21.94']
['ds102', 'sub002', 'M', '22.79']
['ds102', 'sub003', 'M', '19.65']
['ds102', 'sub004', 'M', '25.98']
['ds102', 'sub005', 'M', '23.24']
['ds102', 'sub006', 'M', '23.27']
['ds102', 'sub007', 'D', '34.72']
['ds102', 'sub008', 'D', '22.22']
['ds102', 'sub009', 'M', '22.7']
['ds102', 'sub010', 'D', '25.24']
Writing CSV files¶
Now, we want to write the same data without the first experiment_id column in CSV format to a csv-file. First, let’s delete the first column in the dataset.
data_new = [line[1:] for line in data]
for line in data_new:
print(line)
['sub001', 'F', '21.94']
['sub002', 'M', '22.79']
['sub003', 'M', '19.65']
['sub004', 'M', '25.98']
['sub005', 'M', '23.24']
['sub006', 'M', '23.27']
['sub007', 'D', '34.72']
['sub008', 'D', '22.22']
['sub009', 'M', '22.7']
['sub010', 'D', '25.24']
Now, we first have to open a file again, but this time with writing permissions = 'w'
. After it, we can go through the file and write each line to the new csv-file.
f = open('demographics_new.csv','w') # open a file with writing rights = 'w'
fw = csv.writer(f) # create csv writer
fw.writerows(data_new) # write content to file
f.close() # close file
Lets now check the content of demographics_new.csv
.
!cat demographics_new.csv
sub001,F,21.94
sub002,M,22.79
sub003,M,19.65
sub004,M,25.98
sub005,M,23.24
sub006,M,23.27
sub007,D,34.72
sub008,D,22.22
sub009,M,22.7
sub010,D,25.24
Reading TXT files¶
The reading of txt files is quite similar to the reading of csv-files. The only difference is in the name of the reading function and the formatting that has to be applied to the input or output.
f = open('demographics.txt','r') # open file with reading rights = 'r'
# go through file and trim the new line '\n' at the end
datatxt = [i.splitlines() for i in f.readlines()]
# go through data and split elements in line by tabulators '\t'
datatxt = [i[0].split('\t') for i in datatxt]
f.close() # close file again
for line in datatxt:
print(line)
['ds102', 'sub001', 'F', '21.94']
['ds102', 'sub002', 'M', '22.79']
['ds102', 'sub003', 'M', '19.65']
['ds102', 'sub004', 'M', '25.98']
['ds102', 'sub005', 'M', '23.24']
['ds102', 'sub006', 'M', '23.27']
['ds102', 'sub007', 'D', '34.72']
['ds102', 'sub008', 'D', '22.22']
['ds102', 'sub009', 'M', '22.7']
['ds102', 'sub010', 'D', '25.24']
Writing TXT files¶
The writing of txt files is as follows:
f = open('demograhics_new.txt', 'w') # open file with writing rights = 'w'
datatxt_new = [line[1:] for line in datatxt] # delete first column of array
# Go through datatxt array and write each line with specific format to file
for line in datatxt_new:
f.write("%s\t%s\t%s\n"%(line[0],line[1],line[2]))
f.close() # close file
with open
¶
The previous methods to open or write a file always required that you also close the file again with the close()
function. If you don’t want to worry about this, you can also use the with open
approach. For example:
with open('demographics.txt','r') as f:
datatxt = [i.splitlines() for i in f.readlines()]
datatxt = [i[0].split('\t') for i in datatxt]
for line in datatxt:
print(line)
['ds102', 'sub001', 'F', '21.94']
['ds102', 'sub002', 'M', '22.79']
['ds102', 'sub003', 'M', '19.65']
['ds102', 'sub004', 'M', '25.98']
['ds102', 'sub005', 'M', '23.24']
['ds102', 'sub006', 'M', '23.27']
['ds102', 'sub007', 'D', '34.72']
['ds102', 'sub008', 'D', '22.22']
['ds102', 'sub009', 'M', '22.7']
['ds102', 'sub010', 'D', '25.24']
File modes¶
Read-only:
r
Write-only:
w
(Create a new file or overwrite existing file)Append a file:
a
Read and Write:
r+
Binary mode:
b
(Use for binary files, especially on Windows)
Why do data science in Python?¶
Easy to learn¶
Readable, explicit syntax
Most packages are very well documented
e.g.,
scikit-learn
’s documentation is widely held up as a model
A huge number of tutorials, guides, and other educational materials
Comprehensive standard library¶
The Python standard library contains a huge number of high-quality modules
When in doubt, check the standard library first before you write your own tools!
For example:
os
: operating system toolsre
: regular expressionscollections
: useful data structuresmultiprocessing
: simple parallelization toolspickle
: serializationjson
: reading and writing JSON
Exceptional external libraries¶
Python has very good (often best-in-class) external packages for almost everything
Particularly important for data science, which draws on a very broad toolkit
Package management is easy (conda, pip)
Examples:
Web development: flask, Django
Database ORMs: SQLAlchemy, Django ORM (w/ adapters for all major DBs)
Scraping/parsing text/markup: beautifulsoup, scrapy
Natural language processing (NLP): nltk, gensim, textblob
Numerical computation and data analysis: numpy, scipy, pandas, xarray
Machine learning: scikit-learn, Tensorflow, keras
Image processing: pillow, scikit-image, OpenCV
Plotting: matplotlib, seaborn, altair, ggplot, Bokeh
GUI development: pyQT, wxPython
Testing: py.test
Etc. etc. etc.
(Relatively) good performance¶
Python is a high-level dynamic language—this comes at a performance cost
For many (not all!) data scientists, performance is irrelevant most of the time
In general, the less Python code you write yourself, the better your performance will be
Much of the standard library consists of Python interfaces to C functions
Numpy, scikit-learn, etc. all rely heavily on C/C++ or Fortran
Python vs. other data science languages¶
Python competes for mind share with many other languages
Most notably, R
To a lesser extent, Matlab, Mathematica, SAS, Julia, Java, Scala, etc.
R¶
R is dominant in traditional statistics and some fields of science
Has attracted many SAS, SPSS, and Stata users
Exceptional statistics support; hundreds of best-in-class libraries
Designed to make data analysis and visualization as easy as possible
Slow
Language quirks drive many experienced software developers crazy
Less support for most things non-data-related
MATLAB¶
A proprietary numerical computing language widely used by engineers
Good performance and very active development, but expensive
Closed ecosystem, relatively few third-party libraries
There is an open-source port (Octave)
Not suitable for use as a general-purpose language
So, why Python?¶
Why choose Python over other languages?
Arguably none of these offers the same combination of readability, flexibility, libraries, and performance
Python is sometimes described as “the second best language for everything”
Doesn’t mean you should always use Python
Depends on your needs, community, etc.
You can have your cake and eat it!¶
Many languages—particularly R—now interface seamlessly with Python
You can work primarily in Python, fall back on R when you need it (or vice versa)
The best of all possible worlds?
The core Python data science stack¶
The Python ecosystem contains tens of thousands of packages
Several are very widely used in data science applications:
Jupyter: interactive notebooks
Numpy: numerical computing in Python
pandas: data structures for Python
Scipy: scientific Python tools
Matplotlib: plotting in Python
scikit-learn: machine learning in Python
We’ll cover the first three very briefly here
Other tutorials will go into greater detail on most of the others
The Jupyter notebook¶
“The Jupyter Notebook is a web application that allows you to create and share documents that contain live code, equations, visualizations and explanatory text.”
You can try it online
Supports many different languages
A living document wrapped around a command prompt
Various extensions and widgets
Why Jupyter?¶
An easy way to write and share completely reproducible documents
Combine code, results, and text in one place
You can mix languages
Completely interactive: make a change and see what happens
Execution order matters
Slideshow mode¶
These slides are actually a live Jupyter notebook
We can edit and execute cells on-the-fly
The slideshow extension is installed separately; follow the instructions here
Built-in LaTeX support¶
Highly customizable¶
Custom key bindings
Supports web standards that enable near-limitless customization via JavaScript
All kinds of unofficial extensions
Magic functions¶
Jupyter/IPython includes a number of “magic” commands to make life easier
Support in-line plotting, timing, debugging, calling other languages, etc.